Craig Thorburn - A reinforcement learning account of speech category acquisition
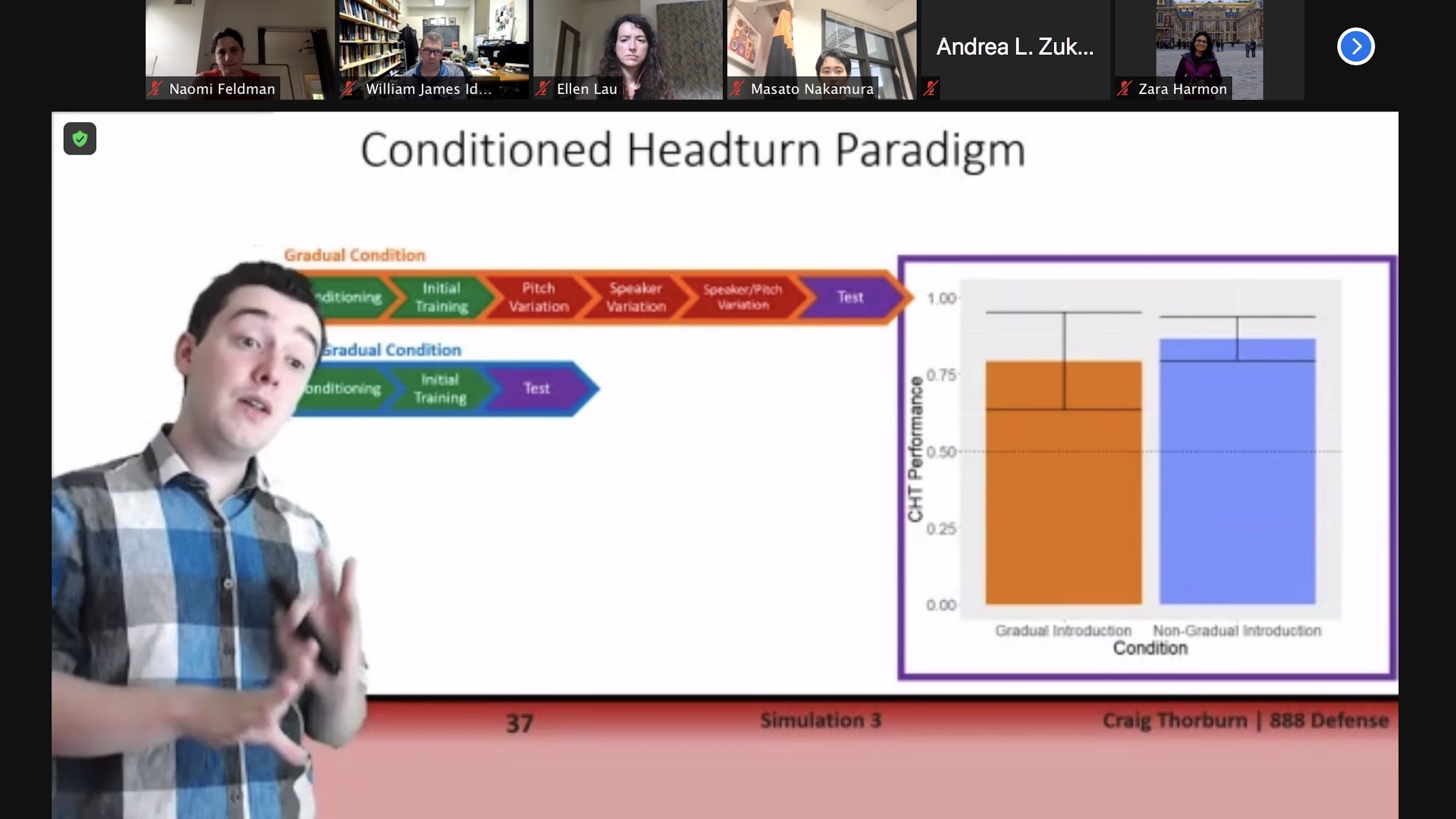
Craig Thorburn - A reinforcement learning account of speech category acquisition
June 8, Craig Thorburn defends his 888, "A reinforcement learning account of speech category acquisition," advised by Naomi Feldman, with a committee also including Ellen Lau and Bill Idsardi. In this work, Craig gives a computational model of experiments where adults learn auditory categories with surprising success, framing it as a case of reinforcement learning. Craig then projects his model onto infant behavior in conditioned head-turn experiments on phonetic learning, and counts it success there as "evidence towards the theory that reinforcement learning is important for category learning across the lifespan."
Introduction
When acquiring a language, one must learn to map a continuous, multidimensional acoustic signal to discrete abstract speech categories. This is an important step which occurs whether learning a first language as an infant or a second language later in life. The complexity of this mapping poses a difficult learning problem, which has been addressed for years through linguistic, neuroscientific, and computational work. Exposure to language at a young age is known to play a vital role in the learning of this process. From as young as 6 months old, infants show effects from language exposure, including a selective ability to discriminate between the sounds of their native language, and an increased ability to distinguish speakers of their own language. While this learning occurs at rapid timescales and without explicit supervision in infants, adults struggle to acquire the speech sounds of a non-native language, and almost never reach native-like ability. A common example to illustrate this phenomenon is the distinction between /r/ and /l/ (Goto, 1971). Although these sounds are distinct in English and a native English speaker will be able to easily distinguish the two sounds, a native Japanese speaker will struggle, as the sounds are not contrastive in their language. Even with much explicit training, Japanese speakers will never reach native-like ability. A particularly effective strategy for learning speech categories as an adult, however, is through implicit learning within a video game paradigm (Wade and Holt, 2005; Gabay et al., 2015; Lim and Holt, 2011). In these experiments, participants play a video game where aliens enter from different sides of the screen. Participants must shoot or capture these aliens and receive a point every time they do so. Auditory tokens presented during the experiment correspond to the aliens’ location and color, and participants show effective learning of these categories. This occurs despite not being informed the auditory categories are relevant for the game. After 5 days of training in this paradigm, adults show as much improvement in the discrimination of non-native speech sounds as 2-4 weeks of explicit training. The effectiveness of learning in this environment naturally leads us to question what algorithm adults could use. In this paper, I model this training paradigm as a reinforcement learning problem, where adults receive rewards for correct actions, and propose that this mechanism could prove essential to effective speech category learning. I provide a precise computational framing of a reinforcement learning approach, not only to adult video game experiments, but also to the conditioned head-turn procedure in infants. The ability of this model to capture both adult and infant learning data provides evidence towards the theory that reinforcement learning is important for category learning across the lifespan. I discuss how this model can be used to answer other important questions within the realm of speech category learning.