Sharon Goldwater / Analyzing representations of self-supervised speech models
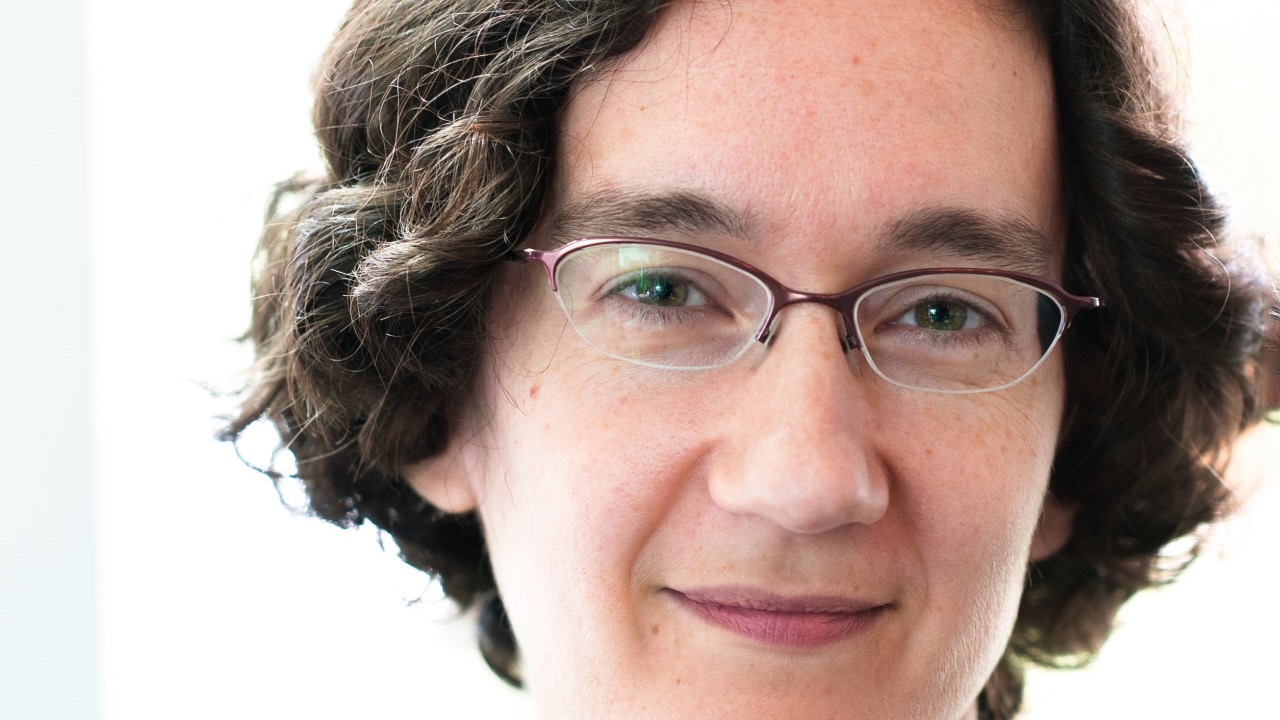
Sharon Goldwater / Analyzing representations of self-supervised speech models
November 10, the Linguistics Colloquium welcomes Sharon Goldwater from Edinburgh University, who will present her research on Bayesian learning, on "Analyzing representations of self-supervised speech models." An abstract follows.
Analyzing representations of self-supervised speech models
Recent advances in speech technology make heavy use of pre-trained models that learn from large quantities of raw (untranscribed) speech, using "self-supervised" (i.e. unsupervised) learning. These models learn to transform the acoustic input into a different representational format that makes supervised learning much easier for tasks such as transcription or even translation. However, what and how speech-relevant information is encoded in these representations is not well understood. I will talk about some work at various stages of completion in which my group is analyzing the structure of these representations, to gain a more systematic understanding of how word-level, phonetic, and speaker information is encoded.