Alum Aaron White wins NSF Early Career Development Award
April 24, 2023
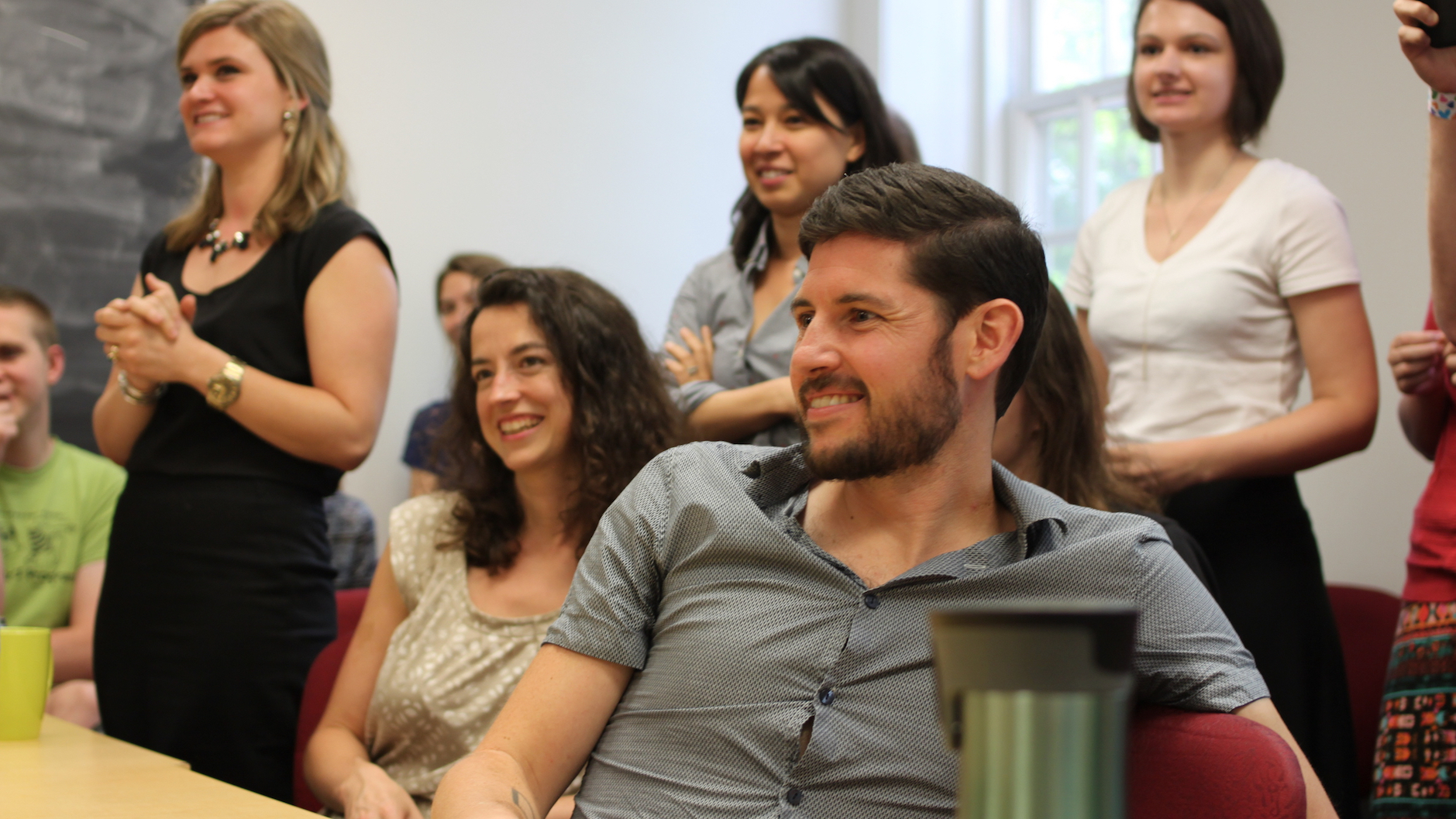
Inducing logical forms for reasoning with artificial intelligence.
Big congratulations to alum Aaron Steven White *15, Assistant Professor at the University of Rochester, who has won support from the NSF's Faculty Early Career Development Program - also known as CAREER - for his project on "Logical Form Induction" (BCS #22371375). The project aims to induce mappings from sentence-representations in AI systems to compositional logical forms that permit models of natural inference (Aaron's abstract is below). The NSF describes CAREER grants as "the most prestigious awards in support of early-career faculty who have the potential to serve as academic role models in research and education and to lead advances in the mission of their department or organization," adding that "activities pursued by early-career faculty should build a firm foundation for a lifetime of leadership in integrating education and research."
At Maryland, Aaron wrote his dissertation on "Information and Incrementality in Syntactic Bootstrapping," aiming "to construct a computational model of syntactic bootstrapping[, and to] use this model to investigate the limits on the amount of information about propositional attitude verb meanings that can be gleaned from syntactic distributions." His supervisors were Valentine Hacquard and Jeff Lidz, who chaired a dissertation committee that also included Philip Resnik and Naomi Feldman.
Logical Form Induction
Artificial intelligence (AI) systems' natural language processing capabilities have made remarkable strides in recent years. Beyond their numerous commercial applications, these advances suggest that AI systems might be powerful tools for deepening our understanding of how humans comprehend natural language. A major obstacle to using them for this purpose is that, while they seem to simulate certain aspects of reasoning by analogy quite well, their capacity to simulate complex logical reasoning shows much room for improvement. This project develops a framework for integrating complex logical reasoning capabilities into the components of AI systems that make their ability to reason by analogy possible. To support the development of this framework, the project builds a large dataset capturing the logical relationships among sentences in three languages by using AI systems to determine which kinds of logical relationships are most useful for improving that system?s own logical reasoning capabilities. Through integration with graduate and undergraduate curricula, the project serves as a vehicle to enhance programming and statistical literacy as well as data collection and data management skills through training with hands-on applications.
The framework integrates logical representations into AI systems by imposing constraints on the sorts of numeric representations that those systems use to make inferences on the basis of some natural language input. These constraints are defined in terms of a mapping from the system's numeric representations of natural language to logical representations. This mapping is learned from scratch and itself constrained (a) to correctly predict inferences that actual speakers of a language make, as captured by the large-scale datasets collected under the project, and (b) to be compositional: the meaning of some piece of language must be predictable from the meanings of its parts.