Laurel, Naomi and Jeff in Cognitive Science
January 28, 2022
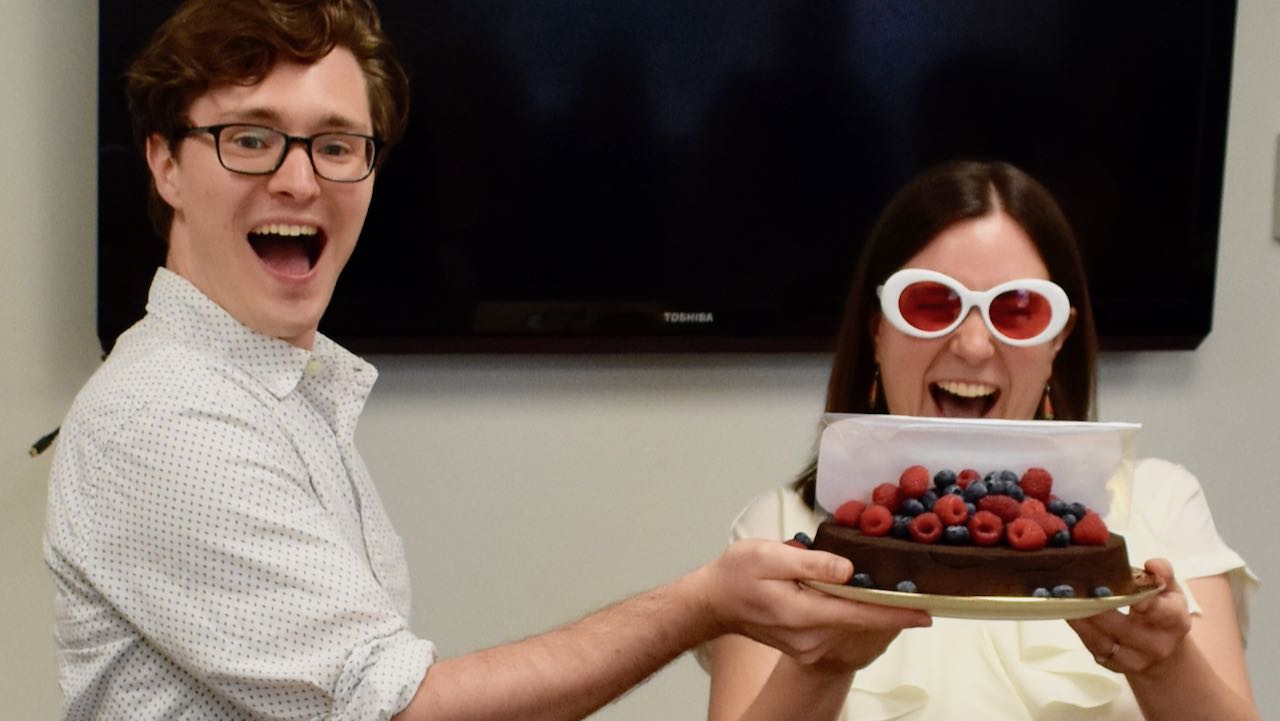
How to avoid learning from misleading data without knowing that it's misleading.
Now out in Cognitive Science, "The Power of Ignoring: Filtering Input for Argument Structure Acquisition," by Laurel Perkins *19, now Assistant Professor of Linguistics at UCLA, with former advisors Naomi Feldman and Jeff Lidz. The article provides a learning model that helps answer a puzzling question: How do young children avoid making bad inferences from data they do not yet represent correctly? For example, how does an infant who does not yet represent wh-dependencies avoid inferring that fix is optionally intransitive, given that it occurs without its 'normal' direct object in questions like What did Laurel fix? The learner cannot know that the data is misleading, prior to representing it correctly. But Laurel shows that a kind of naive humility may provide a way out. She demonstrates computationally that a learner who infers their degree of ignorance, or likelihood of misparsing what it hears, and filters their data accordingly, is able to correctly infer the transitivity for the majority of 50 frequent verbs in child-directed speech.
Abstract
Learning in any domain depends on how the data for learning are represented. In the domain of language acquisition, children’s representations of the speech they hear determine what generalizations they can draw about their target grammar. But these input representations change over development as a function of children’s developing linguistic knowledge, and may be incomplete or inaccurate when children lack the knowledge to parse their input veridically. How does learning succeed in the face of potentially misleading data? We address this issue using the case study of “non-basic” clauses in verb learning. A young infant hearing What did Amy fix? might not recognize that what stands in for the direct object of fix, and might think that fix is occurring without a direct object. We follow a previous proposal that children might filter nonbasic clauses out of the data for learning verb argument structure, but offer a new approach. Instead of assuming that children identify the data to filter in advance, we demonstrate computationally that it is possible for learners to infer a filter on their input without knowing which clauses are nonbasic. We instantiate a learner that considers the possibility that it misparses some of the sentences it hears, and learns to filter out those parsing errors in order to correctly infer transitivity for the majority of 50 frequent verbs in child-directed speech. Our learner offers a novel solution to the problem of learning from immature input representations: Learners may be able to avoid drawing faulty inferences from misleading data by identifying a filter on their input, without knowing in advance what needs to be filtered.