Philip Resnik
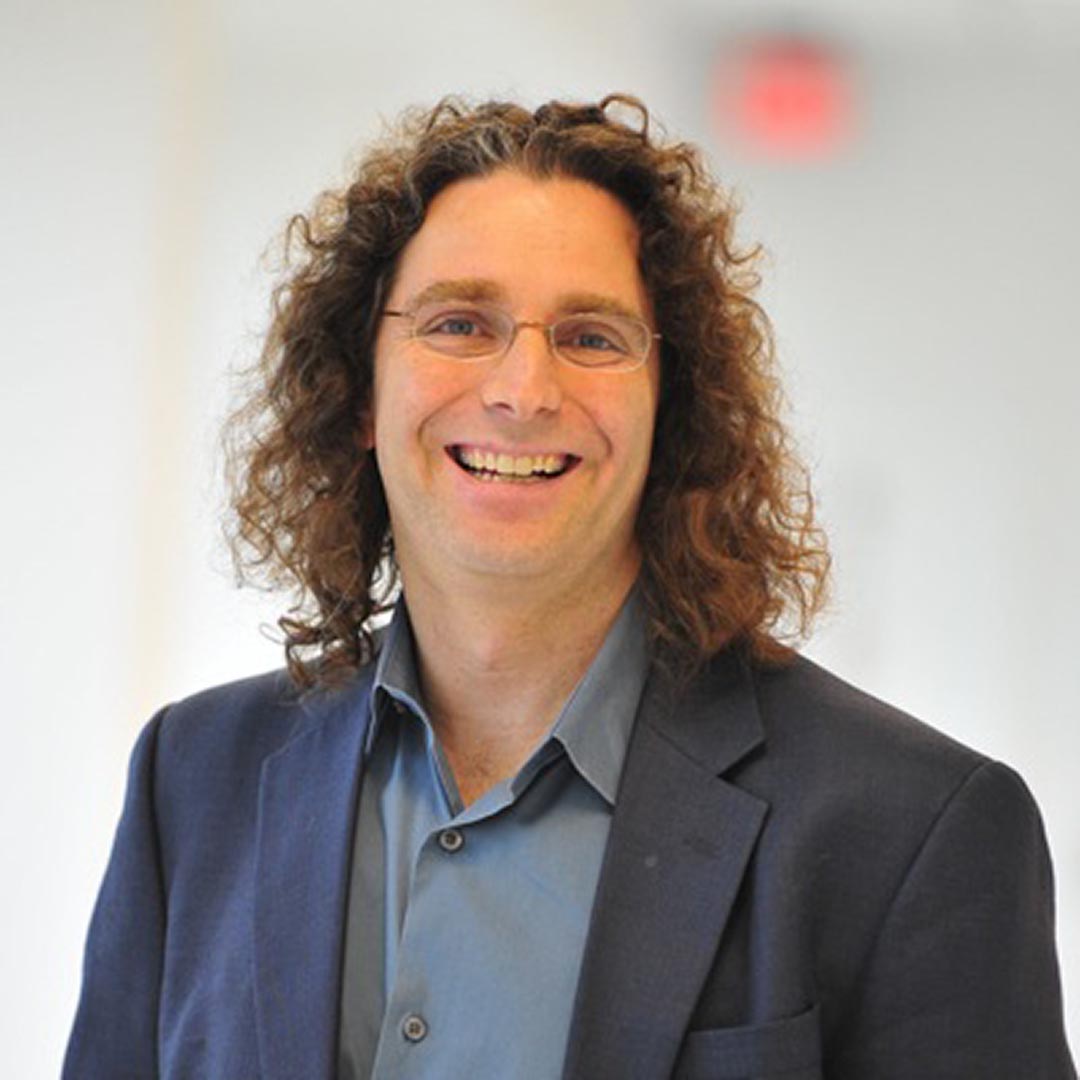
Professor, Linguistics
Member, Maryland Language Science Center
Affiliate Professor, Department of Computer Science
Professor, Institute for Advanced Computer Studies
resnik@umd.edu
1401 C Marie Mount Hall
Get Directions
Research Expertise
Computational Linguistics
Computational Modeling
Publications
Parallel processing in speech perception with local and global representations of linguistic context
MEG evidence for parallel representation of local and global context in speech processing.
Contributor(s): Ellen Lau, Philip Resnik, Shohini BhattasaliNon-ARHU Contributor(s): Christian Brodbeck, Aura Cruz Heredia, Jonathan Simon
Speech processing is highly incremental. It is widely accepted that human listeners continuously use the linguistic context to anticipate upcoming concepts, words, and phonemes. However, previous evidence supports two seemingly contradictory models of how a predictive context is integrated with the bottom-up sensory input: Classic psycholinguistic paradigms suggest a two-stage process, in which acoustic input initially leads to local, context-independent representations, which are then quickly integrated with contextual constraints. This contrasts with the view that the brain constructs a single coherent, unified interpretation of the input, which fully integrates available information across representational hierarchies, and thus uses contextual constraints to modulate even the earliest sensory representations. To distinguish these hypotheses, we tested magnetoencephalography responses to continuous narrative speech for signatures of local and unified predictive models. Results provide evidence that listeners employ both types of models in parallel. Two local context models uniquely predict some part of early neural responses, one based on sublexical phoneme sequences, and one based on the phonemes in the current word alone; at the same time, even early responses to phonemes also reflect a unified model that incorporates sentence level constraints to predict upcoming phonemes. Neural source localization places the anatomical origins of the different predictive models in non-identical parts of the superior temporal lobes bilaterally, with the right hemisphere showing a relative preference for more local models. These results suggest that speech processing recruits both local and unified predictive models in parallel, reconciling previous disparate findings. Parallel models might make the perceptual system more robust, facilitate processing of unexpected inputs, and serve a function in language acquisition.
Using surprisal and fMRI to map the neural bases of broad and local contextual prediction during natural language comprehension
Modeling the influence of local and topical context on processing via an analysis of fMRI time courses during naturalistic listening.
Contributor(s): Philip Resnik, Shohini BhattasaliContext guides comprehenders’ expectations during language processing, and information theoretic surprisal is commonly used as an index of cognitive processing effort. However, prior work using surprisal has considered only within-sentence context, using n-grams, neural language models, or syntactic structure as conditioning context. In this paper, we extend the surprisal approach to use broader topical context, investigating the influence of local and topical context on processing via an analysis of fMRI time courses collected during naturalistic listening. Lexical surprisal calculated from ngram and LSTM language models is used to capture effects of local context; to capture the effects of broader context a new metric based on topic models, topical surprisal, is introduced. We identify distinct patterns of neural activation for lexical surprisal and topical surprisal. These differing neuro-anatomical correlates suggest that local and broad contextual cues during sentence processing recruit different brain regions and that those regions of the language network functionally contribute to processing different dimensions of contextual information during comprehension. More generally, our approach adds to a growing literature using methods from computational linguistics to operationalize and test hypotheses about neuro-cognitive mechanisms in sentence processing.
Automated Topic Model Evaluation Broken? The Incoherence of Coherence
Questioning automatic coherence evaluations for neural topic models.
Contributor(s): Philip ResnikNon-ARHU Contributor(s): Alexander Hoyle, Pranav Goel, Denis Peskov, Andrew Hian-Cheong, Jordan Boyd-Graber
Topic model evaluation, like evaluation of other unsupervised methods, can be contentious. However, the field has coalesced around automated estimates of topic coherence, which rely on the frequency of word co-occurrences in a reference corpus. Recent models relying on neural components surpass classical topic models according to these metrics. At the same time, unlike classical models, the practice of neural topic model evaluation suffers from a validation gap: automatic coherence for neural models has not been validated using human experimentation. In addition, as we show via a meta-analysis of topic modeling literature, there is a substantial standardization gap in the use of automated topic modeling benchmarks. We address both the standardization gap and the validation gap. Using two of the most widely used topic model evaluation datasets, we assess a dominant classical model and two state-of-the-art neural models in a systematic, clearly documented, reproducible way. We use automatic coherence along with the two most widely accepted human judgment tasks, namely, topic rating and word intrusion. Automated evaluation will declare one model significantly different from another when corresponding human evaluations do not, calling into question the validity of fully automatic evaluations independent of human judgments.
Read More about Automated Topic Model Evaluation Broken? The Incoherence of Coherence
Debate Reaction Ideal Points: Political Ideology Measurement Using Real-Time Reaction Data
Estimating an individual's ideology from their real-time reactions to presidential debates.
Contributor(s): Philip ResnikNon-ARHU Contributor(s): Daniel Argyle, Lisa P. Argyle, Vlad Eidelman
Ideal point models have become a powerful tool for defining and measuring the ideology of many kinds of political actors, including legislators, judges, campaign donors, and members of the general public. We extend the application of ideal point models to the public using a novel data source: real-time reactions to statements by candidates in the 2012 presidential debates. Using these reactions as inputs to an ideal point model, we estimate individual-level ideology and evaluate the quality of the measure. Debate reaction ideal points provide a method for estimating a continuous, individual-level measure of ideology that avoids survey response biases, provides better estimates for moderates and the politically unengaged, and reflects the content of salient political discourse relevant to viewers’ attitudes and vote choices. As expected, we find that debate reaction ideal points are more extreme among respondents who strongly identify with a political party, but retain substantial within-party variation. Ideal points are also more extreme among respondents who are more politically interested. Using topical subsets of the debate statements, we find that ideal points in the sample are more moderate for foreign policy than for economic or domestic policy.
A direct comparison of theory-driven and machine learning prediction of suicide: A meta-analysis
Machine learning models are better than models driven by psychological theories in predicting suicidal ideation and suicide attempts.
Contributor(s): Philip ResnikNon-ARHU Contributor(s): Katherine M. Schafer, Grace Kennedy, Austin Gallyer
Theoretically-driven models of suicide have long guided suicidology; however, an approach employing machine learning models has recently emerged in the field. Some have suggested that machine learning models yield improved prediction as compared to theoretical approaches, but to date, this has not been investigated in a systematic manner. The present work directly compares widely researched theories of suicide (i.e., BioSocial, Biological, Ideation-to-Action, and Hopelessness Theories) to machine learning models, comparing the accuracy between the two differing approaches. We conducted literature searches using PubMed, PsycINFO, and Google Scholar, gathering effect sizes from theoretically-relevant constructs and machine learning models. Eligible studies were longitudinal research articles that predicted suicide ideation, attempts, or death published prior to May 1, 2020. 124 studies met inclusion criteria, corresponding to 330 effect sizes. Theoretically-driven models demonstrated suboptimal prediction of ideation (wOR = 2.87; 95% CI, 2.65–3.09; k = 87), attempts (wOR = 1.43; 95% CI, 1.34–1.51; k = 98), and death (wOR = 1.08; 95% CI, 1.01–1.15; k = 78). Generally, Ideation-to-Action (wOR = 2.41, 95% CI = 2.21–2.64, k = 60) outperformed Hopelessness (wOR = 1.83, 95% CI 1.71–1.96, k = 98), Biological (wOR = 1.04; 95% CI .97–1.11, k = 100), and BioSocial (wOR = 1.32, 95% CI 1.11–1.58, k = 6) theories. Machine learning provided superior prediction of ideation (wOR = 13.84; 95% CI, 11.95–16.03; k = 33), attempts (wOR = 99.01; 95% CI, 68.10–142.54; k = 27), and death (wOR = 17.29; 95% CI, 12.85–23.27; k = 7). Findings from our study indicated that across all theoretically-driven models, prediction of suicide-related outcomes was suboptimal. Notably, among theories of suicide, theories within the Ideation-to-Action framework provided the most accurate prediction of suicide-related outcomes. When compared to theoretically-driven models, machine learning models provided superior prediction of suicide ideation, attempts, and death.
MonoTrans2: A New Human Computation System to Support Monolingual Translation
A new user interface to support monolingual translation, by people who speak only the source or target language and not both.
Contributor(s): Philip ResnikNon-ARHU Contributor(s): Chang Hu, Benjamin Bedersen, Philip Resnik, Yakov Kronrod