Naomi Feldman
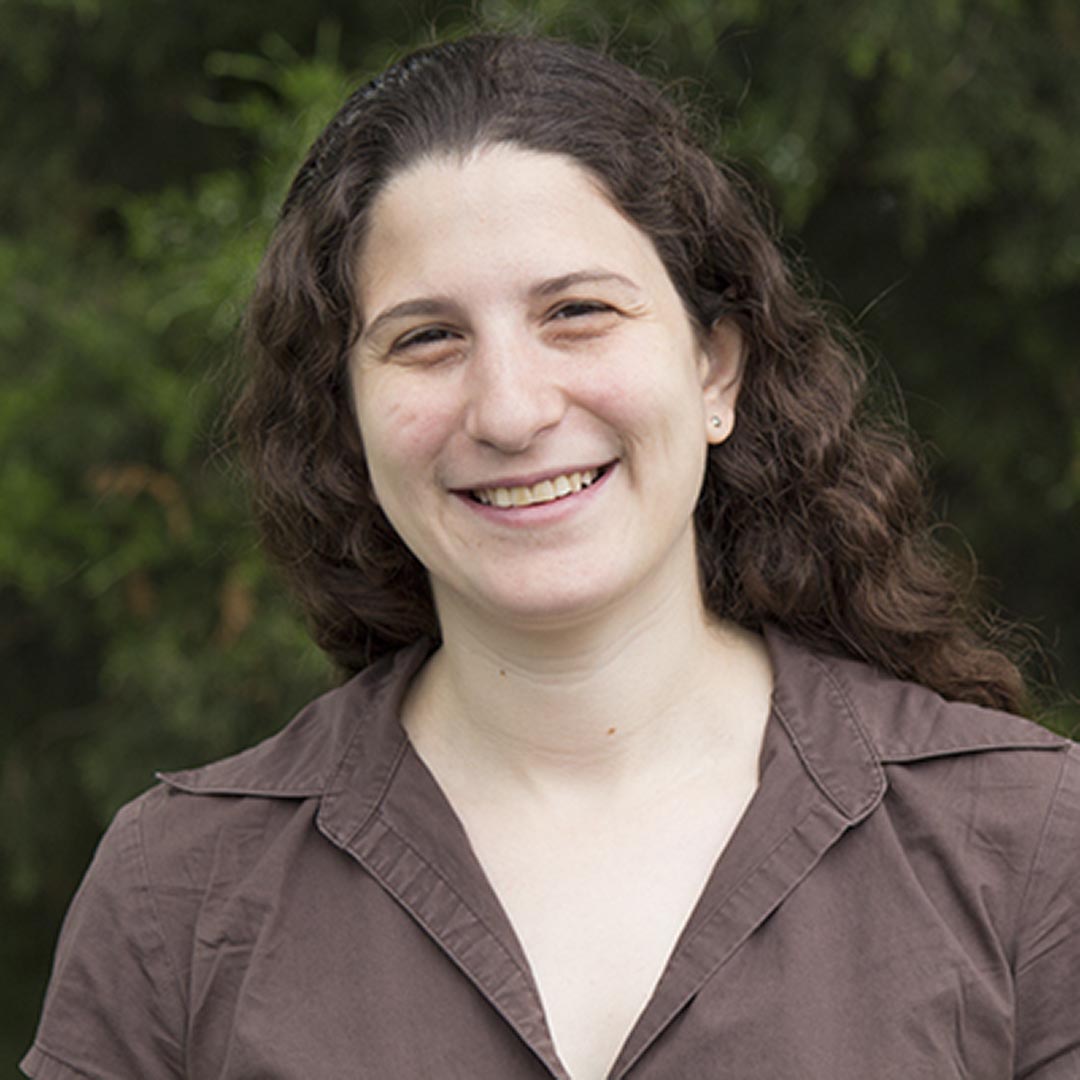
Professor, Linguistics
Member, Maryland Language Science Center
Professor, Institute for Advanced Computer Studies
(301) 405-5800nhf@umd.edu
1413 A Marie Mount Hall
Get Directions
Research Expertise
Computational Linguistics
Computational Modeling
Language Acquisition
Phonology
Publications
Language Discrimination May Not Rely on Rhythm: A Computational Study
Challenging the relationship between rhythm and language discrimination in infancy.
Contributor(s): Leslie Ruolan Li, Naomi FeldmanNon-ARHU Contributor(s): Abi Aboelata (UMD), Thomas Schatz (Marseilles)
It has long been assumed that infants’ ability to discriminate between languages stems from their sensitivity to speech rhythm, i.e., organized temporal structure of vowels and consonants in a language. However, the relationship between speech rhythm and language discrimination has not been directly demonstrated. Here, we use computational modeling and train models of speech perception with and without access to information about rhythm. We test these models on language discrimination, and find that access to rhythm does not affect the success of the model in replicating infant language discrimination results. Our findings challenge the relationship between rhythm and language discrimination,
Read More about Language Discrimination May Not Rely on Rhythm: A Computational Study
Naturalistic speech supports distributional learning across contexts
Infants can learn what acoustic dimensions contrastive by attending to phonetic context.
Contributor(s): Naomi FeldmanNon-ARHU Contributor(s): Kasia Hitczenko *19
At birth, infants discriminate most of the sounds of the world’s languages, but by age 1, infants become language-specific listeners. This has generally been taken as evidence that infants have learned which acoustic dimensions are contrastive, or useful for distinguishing among the sounds of their language(s), and have begun focusing primarily on those dimensions when perceiving speech. However, speech is highly variable, with different sounds overlapping substantially in their acoustics, and after decades of research, we still do not know what aspects of the speech signal allow infants to differentiate contrastive from noncontrastive dimensions. Here we show that infants could learn which acoustic dimensions of their language are contrastive, despite the high acoustic variability. Our account is based on the cross-linguistic fact that even sounds that overlap in their acoustics differ in the contexts they occur in. We predict that this should leave a signal that infants can pick up on and show that acoustic distributions indeed vary more by context along contrastive dimensions compared with noncontrastive dimensions. By establishing this difference, we provide a potential answer to how infants learn about sound contrasts, a question whose answer in natural learning environments has remained elusive.
Read More about Naturalistic speech supports distributional learning across contexts
The Power of Ignoring: Filtering Input for Argument Structure Acquisition
How to avoid learning from misleading data by identifying a filter without knowing what to filter.
Contributor(s): Naomi Feldman, Jeffrey LidzNon-ARHU Contributor(s): Laurel Perkins *19 (UCLA)
Learning in any domain depends on how the data for learning are represented. In the domain of language acquisition, children’s representations of the speech they hear determine what generalizations they can draw about their target grammar. But these input representations change over development asa function of children’s developing linguistic knowledge, and may be incomplete or inaccurate when children lack the knowledge to parse their input veridically. How does learning succeed in the face of potentially misleading data? We address this issue using the case study of “non-basic” clauses inverb learning. A young infant hearing What did Amy fix? might not recognize that what stands in for the direct object of fix, and might think that fix is occurring without a direct object. We follow a previous proposal that children might filter nonbasic clauses out of the data for learning verb argument structure, but offer a new approach. Instead of assuming that children identify the data to filter ina dvance, we demonstrate computationally that it is possible for learners to infer a filter on their input without knowing which clauses are nonbasic. We instantiate a learner that considers the possibility that it misparses some of the sentences it hears, and learns to filter out those parsing errors in order to correctly infer transitivity for the majority of 50 frequent verbs in child-directed speech. Our learner offers a novel solution to the problem of learning from immature input representations: Learners maybe able to avoid drawing faulty inferences from misleading data by identifying a filter on their input,without knowing in advance what needs to be filtered.
Read More about The Power of Ignoring: Filtering Input for Argument Structure Acquisition
Informativity, topicality, and speech cost: comparing models of speakers’ choices of referring expressions
Is use of a pronoun motivated by topicality or efficiency?
Contributor(s): Naomi FeldmanNon-ARHU Contributor(s): Naho Orita *15 (Tokyo University of Science)
This study formalizes and compares two major hypotheses in speakers’ choices of referring expressions: the topicality model that chooses a form based on the topicality of the referent, and the rational model that chooses a form based on the informativity of the form and its speech cost. Simulations suggest that both the topicality of the referent and the informativity of the word are important to consider in speakers’ choices of reference forms, while a speech cost metric that prefers shorter forms may not be.
Social inference may guide early lexical learning
Assessment of knowledgeability and group membership influences infant word learning.
Contributor(s): Naomi Feldman, William IdsardiNon-ARHU Contributor(s): Alayo Tripp *19
We incorporate social reasoning about groups of informants into a model of word learning, and show that the model accounts for infant looking behavior in tasks of both word learning and recognition. Simulation 1 models an experiment where 16-month-old infants saw familiar objects labeled either correctly or incorrectly, by either adults or audio talkers. Simulation 2 reinterprets puzzling data from the Switch task, an audiovisual habituation procedure wherein infants are tested on familiarized associations between novel objects and labels. Eight-month-olds outperform 14-month-olds on the Switch task when required to distinguish labels that are minimal pairs (e.g., “buk” and “puk”), but 14-month-olds' performance is improved by habituation stimuli featuring multiple talkers. Our modeling results support the hypothesis that beliefs about knowledgeability and group membership guide infant looking behavior in both tasks. These results show that social and linguistic development interact in non-trivial ways, and that social categorization findings in developmental psychology could have substantial implications for understanding linguistic development in realistic settings where talkers vary according to observable features correlated with social groupings, including linguistic, ethnic, and gendered groups.
Read More about Social inference may guide early lexical learning
Japanese children's knowledge of the locality of "zibun" and "kare"
Initial errors in the acquisition of the Japanese local- or long-distance anaphor "zibun."
Contributor(s): Jeffrey Lidz, Naomi FeldmanNon-ARHU Contributor(s): Naho Orita *15, Hajime Ono *06
Although the Japanese reflexive zibun can be bound both locally and across clause boundaries, the third-person pronoun kare cannot take a local antecedent. These are properties that children need to learn about their language, but we show that the direct evidence of the binding possibilities of zibun is sparse and the evidence of kare is absent in speech to children, leading us to ask about children’s knowledge. We show that children, unlike adults, incorrectly reject the long-distance antecedent for zibun, and while being able to access this antecedent for a non-local pronoun kare, they consistently reject the local antecedent for this pronoun. These results suggest that children’s lack of matrix readings for zibun is not due to their understanding of discourse context but the properties of their language understanding.
Read More about Japanese children's knowledge of the locality of "zibun" and "kare"
Modeling the learning of the Person Case Constraint
Adam Liter and Naomi Feldman show that the Person Case Constraint can be learned on the basis of significantly less data, if the constraint is represented in terms of feature bundles.
Contributor(s): Adam Liter, Naomi FeldmanMany domains of linguistic research posit feature bundles as an explanation for various phenomena. Such hypotheses are often evaluated on their simplicity (or parsimony). We take a complementary approach. Specifically, we evaluate different hypotheses about the representation of person features in syntax on the basis of their implications for learning the Person Case Constraint (PCC). The PCC refers to a phenomenon where certain combinations of clitics (pronominal bound morphemes) are disallowed with ditransitive verbs. We compare a simple theory of the PCC, where person features are represented as atomic units, to a feature-based theory of the PCC, where person features are represented as feature bundles. We use Bayesian modeling to compare these theories, using data based on realistic proportions of clitic combinations from child-directed speech. We find that both theories can learn the target grammar given enough data, but that the feature-based theory requires significantly less data, suggesting that developmental trajectories could provide insight into syntactic representations in this domain.
A unified account of categorical effects in phonetic perception
A statistical model that explains both the strong categorical effects in perception of consonants, and the very weak effects in perception of vowels.
Contributor(s): Naomi FeldmanNon-ARHU Contributor(s): Yakov Kronrod, Emily Coppess
Read More about A unified account of categorical effects in phonetic perception
Infant-directed speech is consistent with teaching
Why do we speak differently to infants than to adults? To help answer this question, Naomi Feldman offers a formal theory of phonetic teaching and learning.
Contributor(s): Naomi FeldmanNon-ARHU Contributor(s): Baxter Eaves Jr., Thomas Griffiths, Patrick Shafto
Why discourse affects speakers' choice of referring expressions
A probalistic model of the choice between using a pronoun or some other referring expression.
Contributor(s): Naomi FeldmanNon-ARHU Contributor(s): Naho Orita, Eliana Vornov, Hal Daumé III
A role for the developing lexicon in phonetic category acquisition
Bayesian models and artificial language learning tasks show that infant acquiosition of phonetic categories can be helpfully constrained by feedback from word segmentation.
Contributor(s): Naomi FeldmanNon-ARHU Contributor(s): Thomas Griffiths, Sharon Goldwater, James Morgan
Word-level information influences phonetic learning in adults and infants
How do infants learn the phonetic categories of their language? The words they occur can provide a useful cue, shows Naomi Feldman.
Contributor(s): Naomi FeldmanNon-ARHU Contributor(s): Emily Myers, Katherine White, Thomas Griffiths, James Morgan
The influence of categories on perception: Explaining the perceptual magnet effect as optimal statistical inference
Naomi Feldman develops a Bayesian account of the perceptual magnet effect.
Contributor(s): Naomi FeldmanNon-ARHU Contributor(s): Thomas Griffiths, James Morgan
A variety of studies have demonstrated that organizing stimuli into categories can affect the way the stimuli are perceived. We explore the influence of categories on perception through one such phenomenon, the perceptual magnet effect, in which discriminability between vowels is reduced near prototypical vowel sounds. We present a Bayesian model to explain why this reduced discriminability might occur: It arises as a consequence of optimally solving the statistical problem of perception in noise. In the optimal solution to this problem, listeners’ perception is biased toward phonetic category means because they use knowledge of these categories to guide their inferences about speakers’ target productions. Simulations show that model predictions closely correspond to previously published human data, and novel experimental results provide evidence for the predicted link between perceptual warping and noise. The model unifies several previous accounts of the perceptual magnet effect and provides a framework for exploring categorical effects in other domains.